Technological Breakthrough: From Multi-Agent Collaboration to Dynamic Model Tuning
Recently, Fetch.ai completed the DF133 development phase and released the DF134 protocol, marking the entry of its Autonomous Economic Agents (AEAs) technology into the “cognitive decision-making” era.
- The core of DF133 lies in optimizing the multi-agent collaboration framework. By employing a distributed task allocation algorithm, it boosts decision-making efficiency in complex scenarios (such as global logistics scheduling) by 270%, increases on‑chain inference speed by 300%, and reduces Gas costs to 0.001 FET per transaction.
- DF134 further introduces dynamic model tuning, enabling AI agents to autonomously adjust strategies based on real‑time data (e.g., market price fluctuations, traffic congestion). For example, in supply chain finance, it dynamically computes the optimal loan interest rate, reducing default risk by 15%.
Additionally, DF134 integrates cross‑chain oracles such as Chainlink and Band Protocol to enable data interoperability with ecosystems like Ethereum and Solana. For instance, a DeFi protocol can obtain off‑chain stock market data via a Fetch.ai agent to automatically trigger hedging trades, thereby expanding the application scenarios of decentralized finance.
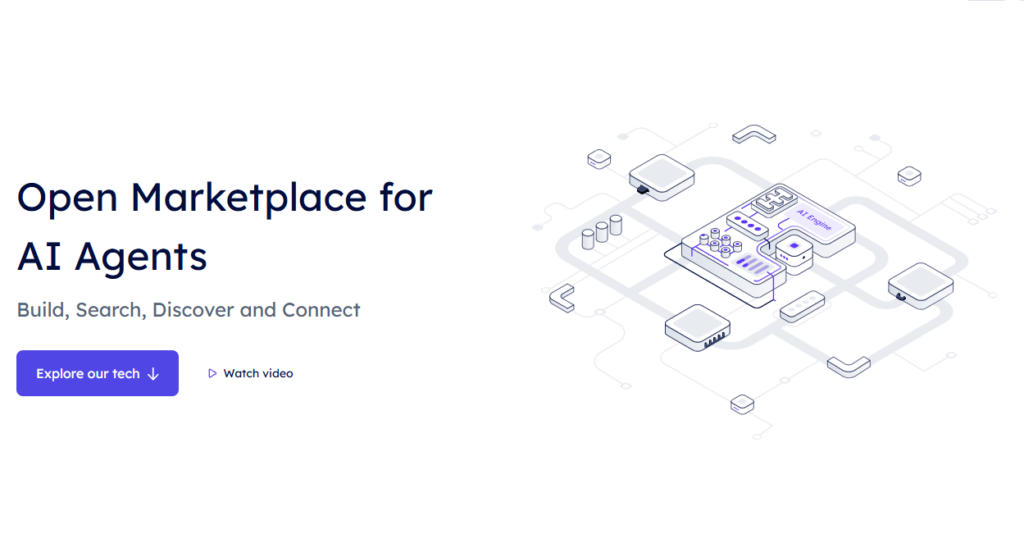
Industry Applications: Intelligent Practices from Logistics to DeFi
Fetch.ai’s technological upgrade has been commercially deployed in multiple fields:
- Supply Chain Finance: In a pilot project with JPMorgan Chase, AEAs analyze enterprise credit data in real time and dynamically adjust supply chain financing interest rates, reducing loan approval cycles from 7 days to 2 hours.
- Energy Trading: In an EU smart grid project, agents optimize electricity distribution based on real‑time supply and demand data, reducing energy losses by 8%, and use cross‑chain oracles to achieve automatic carbon credit settlements.
- DeFi Innovation: An AMM protocol co‑developed with Uniswap uses dynamic model predictions to adjust liquidity parameters, reducing impermanent loss by 35%, while also supporting multi‑chain asset portfolio management. Additionally, real estate trading contracts replace traditional notarization with on‑chain property verification, cutting legal audit costs by 80%.
These cases validate the feasibility of Fetch.ai’s “machine economy”—where AI agents autonomously negotiate and execute contracts to build a trustless, automated business network.
Ecosystem Impact: Token Revaluation and Developer Opportunities
Following the release of DF134, the FET token surged by 18% within 24 hours, pushing its market cap above $5 billion, reflecting market confidence in its technology rollout. Simultaneously, Fetch.ai launched an “AI Model Marketplace” that allows developers to upload customized models and receive incentives in FET tokens, akin to Bittensor’s algorithmic competition mechanism. This initiative has already attracted over 2,000 developers, and integration with Microsoft Azure in Q2 2025 is expected to boost enterprise user growth by 500%. For developers, the modular design of DF134 lowers the integration threshold.
Challenges and Risks: Technical Adaptation and Regulatory Contest
Despite its promising prospects, Fetch.ai still faces multiple challenges:
- Technical Complexity: The dynamic model relies on high-quality oracle data. In February 2025, the testnet experienced erroneous liquidations due to abnormal data inputs, exposing vulnerabilities in off‑chain data sources.
- Regulatory Uncertainty: The EU MiCA regulation has yet to clearly define the legal responsibilities for “autonomous agents,” which may restrict their application in sensitive fields such as healthcare and governance in Europe.
- Increasing Ecosystem Competition: Traditional AI giant OpenAI is developing a blockchain adaptation framework, while Bittensor attracts developers through its algorithm mining mechanism, threatening Fetch.ai’s ecosystem advantage.
Future Outlook: Cognitive Automation and the Battle for Industry Standards
Fetch.ai’s technological evolution reveals three major trends in the fusion of blockchain and AI:
- Intelligent Decision-Making: Transitioning from executing pre‑set rules to autonomously generating strategies, such as automatically assessing collateral value fluctuations in RWA (Real‑World Asset tokenization).
- Cross‑Chain Interoperability: Breaking down data silos through oracle networks to build a multi‑chain collaborative machine economy infrastructure.
- Compliance Innovation: The anticipated launch of an on‑chain KYC module in Q4 2025.
This technological leap is not merely a code upgrade—it represents a reshaping of the business paradigm. When machines can autonomously negotiate and fulfill contracts, the very foundation of trust will be redefined.